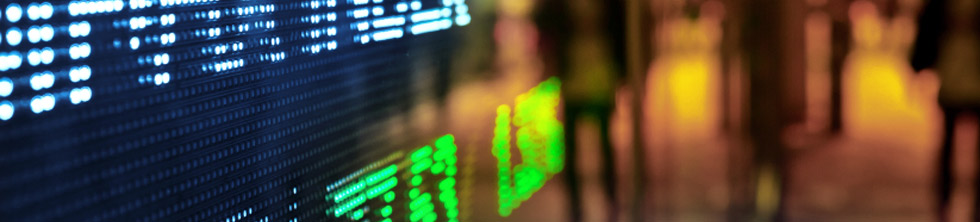
Event
description
The Research Initiative QMI is a research project supported by Université Paris-Dauphine and structured around the following objectives:
1) Promoting quantitative research for asset management;
2) Facilitating the transmission of know-how between academic researchers and asset managers; and
3) Promoting a positive image of quantitative-based asset management through education;
QMI invites applications for PhD professional thesis fellowships. Successful candidates will have demonstrated academic excellence, outstanding potential for creative research, and leadership qualities. QMI thesis fellows will be immersed in an internationally visible, world-class research environment in terms of intellectual and computational resources, also fueled by an extensive visiting program of worldwide academic researchers.
The QMI thesis fellows will also be enrolled in a French-based asset management company, sponsor of QMI. These fellowships will include a net stipend of approximately EUR 1,400/month for three years and generous travel and research funds.
The QMI thesis fellows will also be enrolled in a doctoral school of the Université Paris-Dauphine, with access to intensive short courses in cutting edge areas of the field, Special short courses will be offered in cutting edge research topics by visiting professors. QMI will support yearly summer or winter schools where thesis fellows can meet their future colleagues. All courses will be offered in English and taught by the leading experts in each field – either members of QMI or visiting professors.
The list of thesis project proposals written by members of QMI is provided below. Candidates are invited to contact QMI directly before submitting their application.
Candidates should submit their application to master203@dauphine.psl.eu and arrange for 3 letters of reference to be sent to the same address before the deadline of April 15, 2019.
Agenda
Submission procedure
The deadline for PhD professional thesis applications is April 15, 2019. All applications will be reviewed by the QMI board.
Complete the submission form below and include a resume for each application. These documents must be:
1) In English in PDF format with a maximum size of 3MB;
2) Submitted by e-mail to: master203@dauphine.psl.eu; and
3) Marked ” PhD professional thesis application – IdR FdR QMI”.
Candidates will be notified as to the acceptance of their project by the end of April 2019.
Submission procedure
The deadline for PhD professional thesis applications is April 15, 2019. All applications will be reviewed by the QMI board.
Complete the submission form below and include a resume for each application. These documents must be:
1) In English in PDF format with a maximum size of 3MB;
2) Submitted by e-mail to: master203@dauphine.psl.eu; and
3) Marked ” PhD professional thesis application – IdR FdR QMI”.
Candidates will be notified as to the acceptance of their project by the end of April 2019.
Contact information
Pauline de Saint Quentin – +33 (0)1 72 60 52 34 – master203@dauphine.psl.eu
Université Paris Dauphine
Place du Maréchal de Lattre de Tassigny
75775 Paris Cedex 16 – FRANCE
Submission file
To download the submission file, click here
Venue
List Thesis projects
The QMI committee invites PhD application on topics such as (but not necessarily limited to):
1. Measuring Fund/Index Performance – The Data Challenge
The project aims to build unbiased indices of fund or strategy performance when mandatory disclosure is not the rule, or when information is coming from different data sources. Reporting monthly performance in the asset management industry is, in certain cases, not mandatory (see e.g. hedge funds, private equity funds, risk premia propietary indices, etc.). As a consequence, managers can decide when and where (to what database or databases) they report. Obviously, this choice is not exogenous. For example, new funds/indices can decide to report as soon as they experience one-year (or sometimes even more) of positive returns. The different steps of our research agenda are listed below:
- Analysing fund performance using existing databases: what funds/indices, what is their type, are they reporting to several databases or just to one, … to get a clear idea of the composition of each database;
- Analysing the reasons for their reporting choice;
- Studying the impact of this choice on the performance of a given fund/index in the database. What are their differences? and
- Propose and compute an index representative of fund/index performance.
This research agenda requires, first, the construction of a fund manager database. Understanding the behaviour of fund managers (hedge funds, UCITS, mutual funds, risk premia propietary indices) is key to explain the recent evolution of some markets but also to detect systemic risk factors (regulation implications) and potential conflicts of interest (financial markets law implications). But any analysis of this kind requires collection of information from several sources and to access to big-data-type databases.
2. Measuring and analysing market sentiment indicators for market prediction improvement
The main objective of the project is to use Artifial Intelligence technics (Machine learning, big data …) to capture individual investors’ sentiment, to agregate this information into some market sentiment measures and to analyse if such measures help understanding financial market efficiency. The different steps of our research agenda are the following :
- Identification of the relevant investors so as to form a community of interest.
- Analysis of the information we can get from these investors that could help proposing new market sentiments
- Testing the predicting power of these new measures
This research agenda requires, first, that we get access to a database that gather investor’s individual sentiment. One exemple can be that of Darolles, Le Fol, Le Ny and Monarcha (2018) based on every available tweets collected from every followers of 50 seed chosen accounts since 2007. These accounts, chosen by their alleged notoriety amongst financial medias, are composed of the 25 most important institu-tional accounts according to (WSJ 2016) and the most influential finance peoples as defined by (MarketWatch 2016).
Second, text analysis methods have to be implemented to extract financial contents and to compute some market sentiment indices. Finally, we will use some times series models to see if these measures have a predictive power of return or volatility.
3. Risk premia and investors’ behavior
The main objective of this research is to characterise which proportion of active versus passive investors in a given market or asset class ensure a good behavior of the market/class . On the one hand, as the number of active investors seeking alpha increases, the alpha to share across them decreases to the point that these strategies become unprofitable. On the other one, if there are too many passive investors in the market, the price discovery is decreasing and markets are no more efficient. This research would be based on :
– A model with several populations of investors :
- Active portfolio managers
- Passive portfolio managers
- Noise investors
trading against each other and analyzing what is the proportion of each categories making the system work
– A large database of investors type and strategies
-The estimation and analysis of investors population composition and the link with the average alpha of active strategies
This alpha could be an indicator of the profitability of active strategies per asset type : A small alpha would signal that too many active investors are at play to start and should discourage any active new comer while a large alpha would signal that intering the market following an active strategy should be profitable as the majority of other investors are being passive.
4. Derivatives in AM
Portfolio managers, individual investors and corporates generally use hedging techniques (derivatives) to reduce their exposure to various risks. This is genrally done by adding derivatives instruments to the portfolio.
But derivatives can also be used to build ab exposure to certain risks. The advantage of this approach againt the buy and hold is that the management of the implied risk exposure is by definition much more dynamic. Moreover, this position is also varying with respect to second-order risks such as market volatility or credit risk.
In general, Portfolio allocation with derivatives instruments stays mostly empirical and is essentially based on historical backtests,with a high overfitting risk . The idea of this research is to propose a theoretical background that accounts for derivatives in the portfolio construction process. The objective is to show that the implied dynamic of derivative products can be use to simplify the daily management andreduce the implementation cost of active investment strategies.